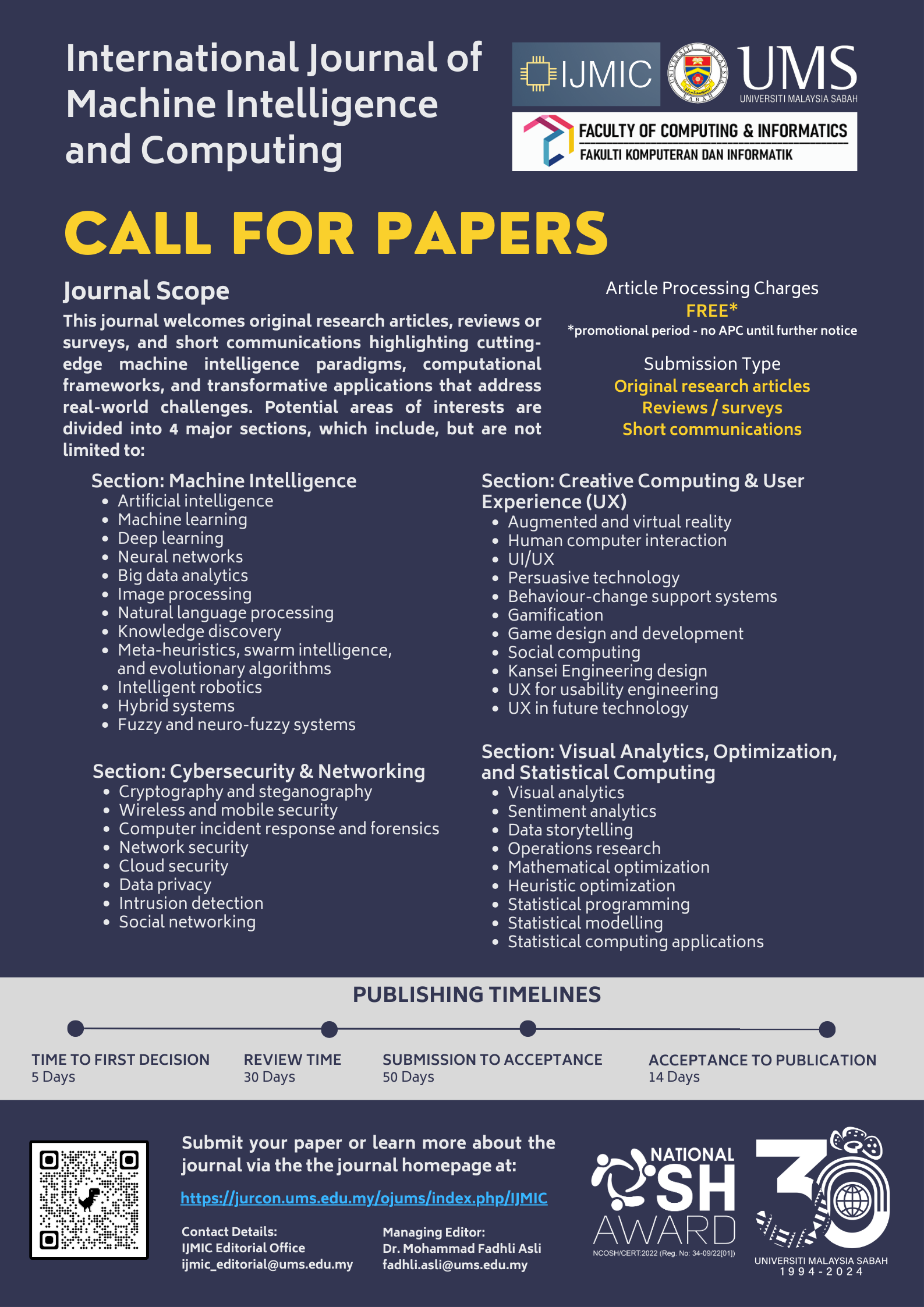
About the Journal
The International Journal of Machine Intelligence and Computing (IJMIC) is an international peer-reviewed publication that focuses on the emerging areas of machine intelligence and computing including the overarching impact of technologies on all aspects of our lives at the societal level.